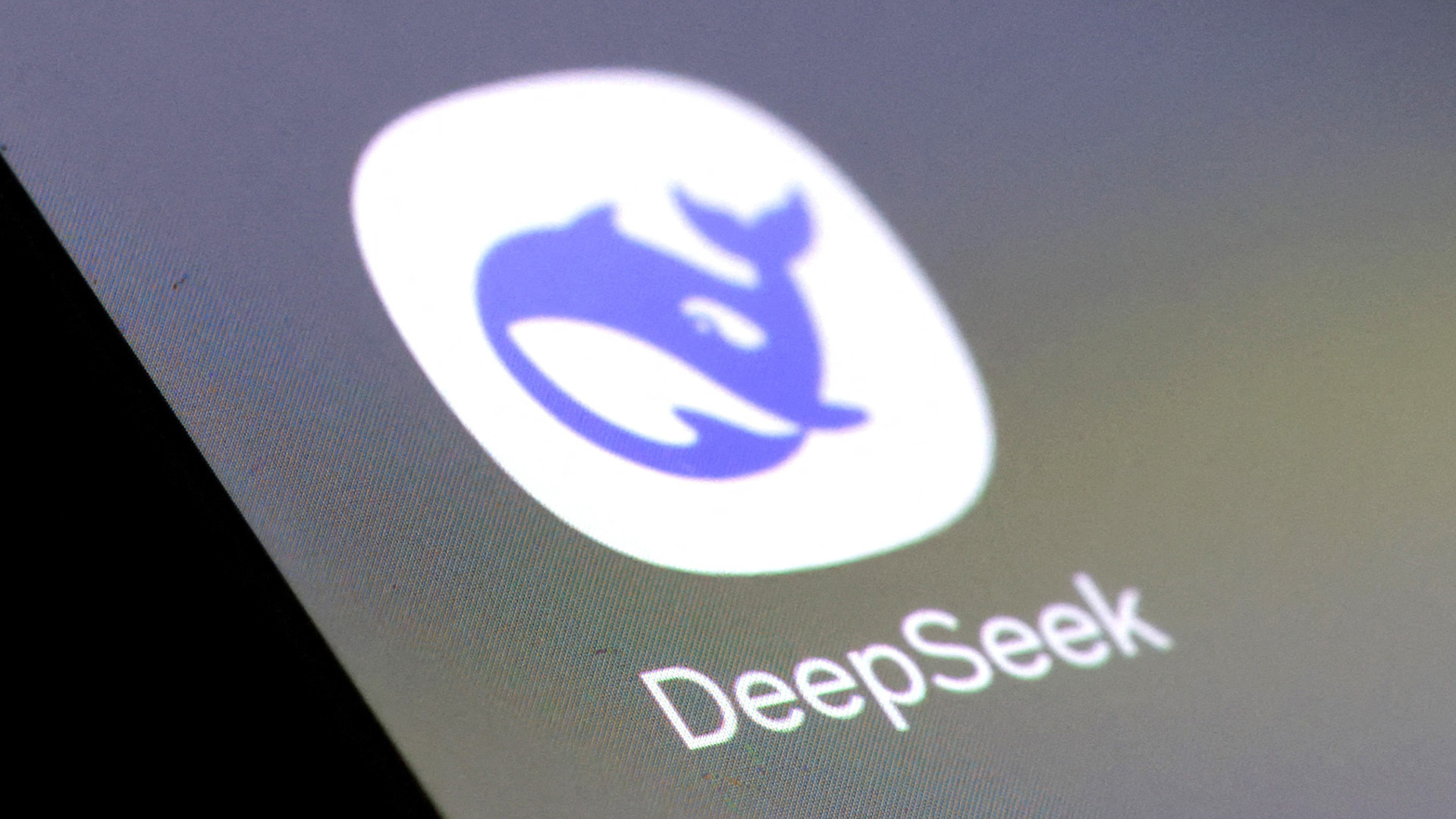
DeepSeek faces federal investigation over how it got its AI chips: Report
By Simone Del Rosario (Business Correspondent), Brent Jabbour (Senior Producer), Emma Stoltzfus (Video Editor)
U.S. officials are reportedly investigating whether Chinese AI startup DeepSeek illegally went around U.S. export controls to score the best Nvidia chips. A Bloomberg report citing people familiar with the matter said the White House and FBI are involved.
Media Landscape
See how news outlets across the political spectrum are covering this story. Learn moreBias Distribution
Left
Untracked Bias
U.S. export controls bar Nvidia from selling the most advanced AI chips to Chinese companies. The U.S. suspects DeepSeek might have gotten around the restriction using third parties in Singapore.
DeepSeek disrupts AI race
During the week of Jan. 26, DeepSeek disrupted the AI race with its chatbot R1. It dethroned OpenAI’s ChatGPT on top of the Apple App Store chart and tanked tech stocks in the process.
Nvidia’s stock is still down about 12% from its share price last Friday. The company shed around $600 billion in market cap on DeepSeek’s arrival. It’s the biggest one-day loss for a U.S. company in history. The higher you climb, the farther you fall.
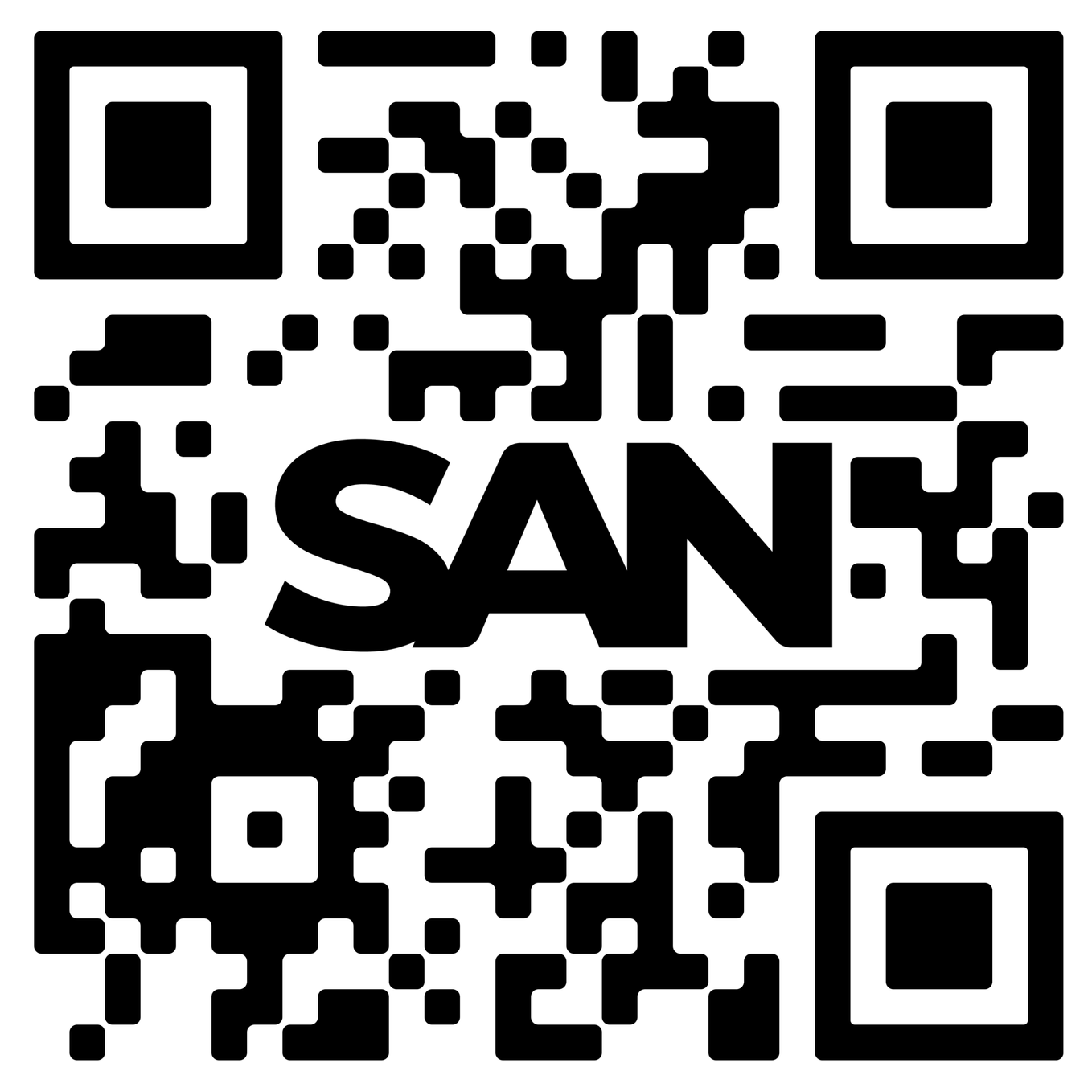
Download the SAN app today to stay up-to-date with Unbiased. Straight Facts™.
Point phone camera here
A big driver to what’s damaging other tech companies is the price DeepSeek claims to have paid to train its high-performing chatbot. According to its research paper, DeepSeek used inferior Nvidia H800 chips to build it and spent just $6 million to train it. But many aren’t buying it.
“I do not believe that DeepSeek was done all above board. That’s nonsense,” said Howard Lutnick, President Donald Trump’s pick for commerce secretary. “They stole things. They broke in. They’ve taken our IP. It’s got to end. And I’m going to be rigorous in our pursuit of restrictions and enforcing those restrictions to keep us in the lead because we must stay in the lead.”
“It doesn’t seem like it’s an obvious red flag that this cannot have been done,” said Tara Javidi, a professor of electrical and computer engineering at UCSD. “Many of us have been doing research in the space, in various aspects of the space, to make the training process cheaper, to make the models smaller, to really think about open-sourcing.”
“When people report the cost of training, they report the cost of training for their last successful attempt where it went through,” she added, indicating DeepSeek’s $6 million total wouldn’t include any previous failed attempts.
Nvidia responds to reports
Since DeepSeek’s arrival, Nvidia has come out saying it doesn’t believe DeepSeek broke any rules. Nvidia told Bloomberg it insists its customers comply with the law and act accordingly if they don’t.
Earlier this month, Nvidia publicly criticized the Biden administration over the export controls put in place. The company said it was looking forward to working with Trump.
Simone Del Rosario: U.S. officials are reportedly investigating whether Chinese AI startup DeepSeek illegally went around U.S. export controls to score the best Nvidia chips.
A Bloomberg report citing people familiar with the matter said the White House and FBI are involved.
U.S. export controls bar Nvidia from selling the most advanced AI chips to Chinese companies. The U.S. suspects DeepSeek might have gotten around the restriction using third parties in Singapore.
This week, DeepSeek disrupted the AI race with its chatbot R1. It dethroned OpenAI’s ChatGPT on top of the Apple App Store chart and tanked tech stocks in the process.
Nvidia’s stock is still down about 12% from its share price last Friday. The company shed around $600 billion in market cap on DeepSeek’s arrival. It’s the biggest one-day loss for a U.S. company in history. The higher you climb, the farther you fall.
A big driver to what’s damaging other tech companies is the price DeepSeek claims to have paid to train its high-performing chatbot.
According to its research paper, DeepSeek used inferior Nvidia H800 chips to build it and spent just $6 million to train it.
But many aren’t buying it.
Howard Lutnick: I do not believe that DeepSeek was done all above board. That’s nonsense. They stole things. They broke in. They’ve taken our IP. It’s got to end. And I’m going to be rigorous in our pursuit of restrictions and enforcing those restrictions to keep us in the lead, because we must stay in the lead.
Simone Del Rosario: Nvidia publicly criticized the Biden administration over the export controls they put in place. They said they were looking forward to working with President Donald Trump.
Since DeepSeek’s arrival, Nvidia has come out saying they don’t believe DeepSeek broke any rules. Nvidia says they insist their customers comply with the law, and act accordingly if they don’t.
I want to bring in Tara Javidi, Lewak Endowed Chair and Professor of Electrical and Computer Engineering at UCSD. Tara, you’ve read DeepSeek’s research paper. I know it’s a lengthy one. Do you believe that they were able to achieve what they have using the modified chips and as little money as they claim?
Tara Javidi: So thank you for having me. Yes, I’ve been looking at this. So I think there are multiple ways to answer this question. Of course, as an academic researcher, I don’t have investigation possibility of knowing the details of their implementation. But I can tell you that a lot of the components of the study are really pulling together lots of work and innovation that has been in the open research space throughout the years. So they have managed to pull that. So in that aspect, the results are not so surprising in the sense of pulling it together. It doesn’t seem like it’s an obvious red flag that this cannot have been done. Many of us have been doing research in the space, in various aspects of the space, to make the training process cheaper, to make the models smaller, to really think about open-sourcing, maybe possibly some of the larger models and questions of this sort have been thrown around in the research community. And then when people report the cost of training, they report the cost of training for their last successful attempt where it went through. They don’t usually report all those other sort of, I have to stop, something was not right, I have to redo. So those are not reported as it’s never reported in any of these studies and including for the DeepSeq. So it’s a little bit of a complicated story there when we talk about the cost of training and whether a big company that already has a nice secret sauce with the training of large models and they have this sort of long training pipeline. whether they will want to take the same risk and really try these more, these methods that are more researchy and are proposed to do this. And this is sort of definitely a bit of the hallmark of this study and the work that has been put out by DeepSea.
Simone Del Rosario: Well, let me ask you this, how is DeepSeek different from OpenAI’s chat GPT and other language learning models?
Tara Javidi: So I guess the most important fact for many of us in the research community is that it’s a large model that is yet open source. And most of the open source efforts that we have seen previously have been at the smaller, what is called smaller model. I want to emphasize these models are still quite large in terms of the number of parameters. So that’s the one piece that is different is that this model, despite the fact that it’s large, it’s open source. Another fact is that it incorporates many methods, as I was saying, from the research community in terms of trying to make the efficiency of the training much more than classical methods that have been proposed for training these large models. So in that sense, the training process itself has been made much more efficient in their cycle.
Simone Del Rosario: Look, with a lot of attention comes a lot of people poking around. Security researchers are finding DeepSeek to be very vulnerable to malicious attacks. We saw that on Monday when they had to actually stop allowing new users to come on board. They limited it to Chinese phone numbers for a bit. But since then, the subsequent research has shown that it is very vulnerable. Is that the risk of being open source or is there something more here?
Tara Javidi: Yeah, I haven’t followed that precisely, but what I can say is that it’s a combination most probably of the process of training and making a model robust. You usually often try to make it robust by ingesting more data and classical ways of dealing with robustness is actually making sure that you build safeguards and these safeguards require you to really think about constructing data and queries that are adversarial to build that. So it could be a byproduct of trying to be very efficient in the first round. You might miss some of the ability to build these safeguards. Of course, if a model is open source, the real difficulty of, know, the economics of this. It’s a lot of work and effort to build a model. And so when you put it open source, anybody can sort of have access to the model to fine tune it, to train it and use it for other. So there are two levels of robustness issues that comes in. one is that your weights are known and the source, the model is known. So the source is available for people to sort of build other things. And that’s one form of lack of robustness. And the other one is sort of safeguarding it against jail breaks and like, you know, getting it to do things that you didn’t mean to build into that.
Simone Del Rosario: So with all of that in mind, do you think that DeepSeek is a worthy competitor in this space right now?
Tara Javidi: In engineering, usually when when the first study that proves something that was imagined to be plausible, yet nobody was doing it, when when that happens, it sort of gives this sense what is doable or what is plausible, sort of brings that. It has definitely, for me, it has been a sort of a point of reference that looking at this now I can be more forceful talking to, for example, federal agencies and really thinking about more support for this kind of foundational research and academic institution. This is a good possibility study to say this is possible and it’s not something that we only need very established methods. That’s the only way that works. It opens the door for a lot of fundamental research at universities to be gaining attention. So in that sense, for academics, this has been a really interesting study to pay attention to.
Simone Del Rosario: Yeah, it opens it up beyond saying, well, only a Microsoft or a Meta or an OpenAI is able to develop something like this.
Tara Javidi: Yeah, and also only the sort of more robust and economically certain paths of doing this kind of model training is the only way to do. As I said, as I was trying and I don’t know if I did a good job as I was trying to explain at the beginning is that when you are a company, you really have you have an obligation really to be picking, you know, sort of the risks that you are taking in a much more calculated way than if you’re doing research at a university. So that has been a major sort of question that we do open in the open research community. We do advocate certain ways of training to modify the understood ways to enable for more efficient training for smaller models for compression and so on and so forth. But we don’t expect that large companies to just jump on that research and immediately do it because of the financial implications. So I think in that sense this is an interesting study for those of us who have been in the research community because it validates, at least on the surface, validates a lot of the sort of open, the kind of questions that have been in the public domain in the research space.
Media Landscape
See how news outlets across the political spectrum are covering this story. Learn moreBias Distribution
Left
Untracked Bias
Straight to your inbox.
By entering your email, you agree to the Terms & Conditions and acknowledge the Privacy Policy.
MOST POPULAR
-
DVIDS
Judge blocks Venezuelan migrants from being sent to Guantánamo Bay
Watch 1:03Yesterday -
AP Images
Trump to announce new 25% tariffs on steel, aluminum
Watch 9:25Yesterday -
Getty Images
Philadelphia Eagles win Super Bowl 59, dominate Kansas City Chiefs, 40-22
ReadSunday -
Getty Images
Eagles dominating Chiefs through first half of Super Bowl 59, lead 24-0
ReadSunday